As artificial intelligence (AI) continues to advance and integrate into various aspects of daily life, addressing the ethical considerations surrounding its development and deployment becomes increasingly crucial. Ensuring that AI systems are designed and used responsibly is essential to building trust and safeguarding human rights. This article explores the key ethical issues in AI and provides insights into how they can be managed.
1. Bias and Fairness
A. Sources of Bias
- Training Data: AI systems learn from data, and if the data contains biases, the AI can perpetuate or even amplify these biases.
- Examples: Biases in recruitment algorithms that favor certain demographic groups over others, or facial recognition systems that perform less accurately for minority groups.
B. Addressing Bias
- Diverse Data Sets: Ensuring that training data is representative of diverse populations and situations can help reduce bias.
- Regular Audits: Implementing regular audits and reviews of AI systems to detect and mitigate biases.
2. Privacy and Data Protection
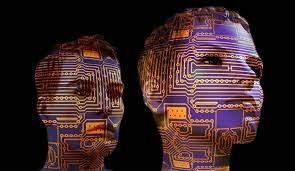
A. Data Collection and Use
- Personal Data: AI systems often require large amounts of personal data, raising concerns about how this data is collected, stored, and used.
- Examples: Health data in AI-driven health applications or personal information in AI-powered recommendation systems.
B. Ensuring Privacy
- Data Anonymization: Techniques like anonymization and pseudonymization can help protect individuals’ privacy while using their data.
- Consent and Transparency: Providing clear information about data collection practices and obtaining informed consent from users.
3. Transparency and Explainability
A. Understanding AI Decisions
- Black-Box Problem: Many AI systems, particularly those using deep learning, operate as “black boxes,” making it difficult to understand how decisions are made.
- Examples: AI-driven financial decisions or legal judgments that impact individuals’ lives.
B. Enhancing Transparency
- Explainable AI (XAI): Developing AI systems that provide clear explanations for their decisions and actions.
- User Communication: Ensuring that users understand how AI systems work and how their data is being used.
4. Accountability and Responsibility
A. Assigning Responsibility
- Liability: Determining who is responsible when an AI system causes harm or makes an erroneous decision.
- Examples: Accountability in autonomous vehicles accidents or financial losses due to flawed AI trading algorithms.
B. Implementing Accountability
- Clear Guidelines: Establishing clear guidelines for accountability and ensuring that stakeholders are aware of their responsibilities.
- Regulatory Oversight: Encouraging regulatory bodies to oversee and enforce accountability in AI systems.
5. Impact on Employment
A. Job Displacement
- Automation: AI and automation technologies can lead to job displacement in certain industries.
- Examples: Manufacturing jobs replaced by AI-driven robots or administrative tasks automated by AI systems.
B. Mitigating Impact
- Reskilling Programs: Providing reskilling and upskilling opportunities for workers affected by AI and automation.
- Job Creation: Fostering the creation of new job roles and opportunities that arise from the advancement of AI technologies.
6. Ethical AI Design and Development
A. Designing for Ethics
- Ethical Principles: Incorporating ethical principles into the design and development of AI systems, such as fairness, transparency, and respect for human rights.
- Examples: Ethical guidelines for AI research and development organizations or AI ethics boards.
B. Collaboration and Engagement
- Stakeholder Involvement: Engaging diverse stakeholders, including ethicists, policymakers, and the public, in the development process to ensure broad perspectives are considered.
- Ethical Reviews: Conducting ethical reviews and impact assessments during the development of AI systems.
7. Long-Term Societal Impact
A. AI and Human Rights
- Rights Considerations: Ensuring that AI systems respect and uphold fundamental human rights, such as privacy, freedom of expression, and non-discrimination.
- Examples: AI applications in surveillance or social credit systems that may infringe on individual rights.
B. Future Implications
- Sustainable Development: Considering the long-term societal implications of AI and striving for responsible and sustainable development practices.
- Global Cooperation: Promoting international cooperation to address global ethical challenges associated with AI technologies.
Conclusion
Ethical considerations in AI development and deployment are vital to ensuring that AI technologies benefit society while minimizing harm. Addressing issues such as bias, privacy, transparency, accountability, and societal impact requires a collaborative approach involving developers, policymakers, and the public. By prioritizing ethical principles and actively working to address challenges, we can build AI systems that are fair, secure, and aligned with human values, ultimately fostering trust and advancing the responsible use of AI.